The future of commercial real estate isn’t about following trends;
it's about setting them. In a market where every decision counts, it’s the VTS mission to arm CRE professionals—owners, brokers, and operators—with the kind of real-time insights that drive smarter, faster, and more profitable decisions. This is why AI is a cornerstone of our product strategy. The success of our customers demands nothing less.
In this article, we’ll dive into how we’re evolving beyond traditional real estate tech by integrating the latest advancements in AI and machine learning. Using cutting-edge tools and techniques like natural language processing, network science, statistics, and transformers, we’re combining data from thousands of sources to give you a clear, real-time, and actionable view of the CRE market. This is more than just a new way to look at data—it’s a new way to lead in commercial real estate.
“The revolution hasn’t happened yet”
Almost all large industries from travel to finance have been completely turned upside down by data and machine learning. But real estate always seems one step away from fully being “disrupted” by technology.
In fact, it’s still quite a few years behind. Whereas most financial markets have centralized, digitized reporting and analysis, most CRE transactions and the details within them have been scattered across various digital applications, news articles, or buried in an excel spreadsheet at a brokerage firm. If you wanted to learn why a particular office building stood empty for 17 months while the office building adjacent was 100% occupied, you might call a broker, tour the space to compare interior fit-outs, or query third-party data to understand leasing activity in the particular submarket.
This is because the industry has remained very fragmented, and there’s been real professional inertia to maintain opacity. Matt Levine of Bloomberg states that buildings are like bonds: “there are a lot of them, they’re all different, they each have idiosyncratic weird issues, the trade size tends to be in the eight figures, no individual building trades all that often”, and that the facts about them are subjective, often not “that computer legible”.
For these reasons and more, at VTS, we believe AI’s biggest impact within CRE will not come from models that draft emails or provide rote answers to empirical questions. It’ll come from far greater challenges associated with analyzing data streams with higher levels of abstraction, and empowering professionals to understand “facts” about the real world.
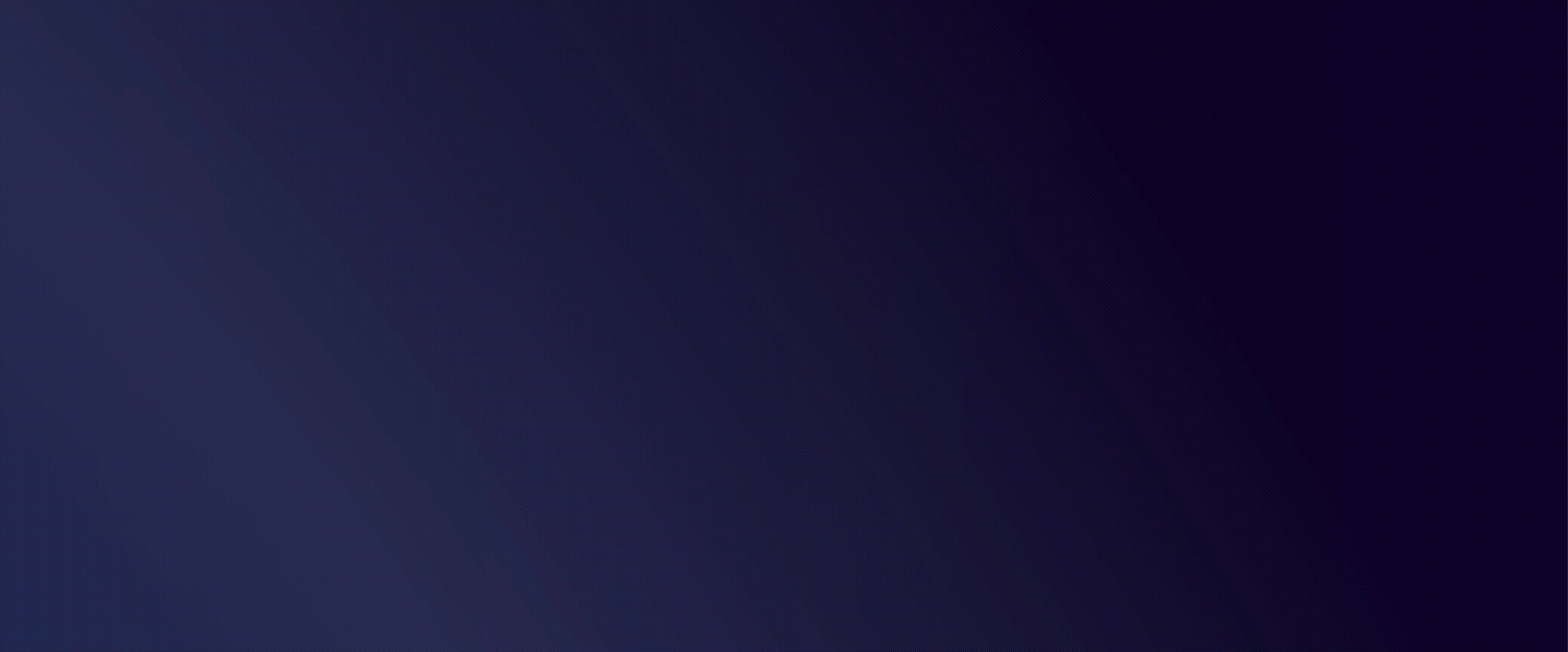
Among several capabilities, our models are trained to make real-world connections between
tenant companies, giving owners a lens into real-time demand trends for office space
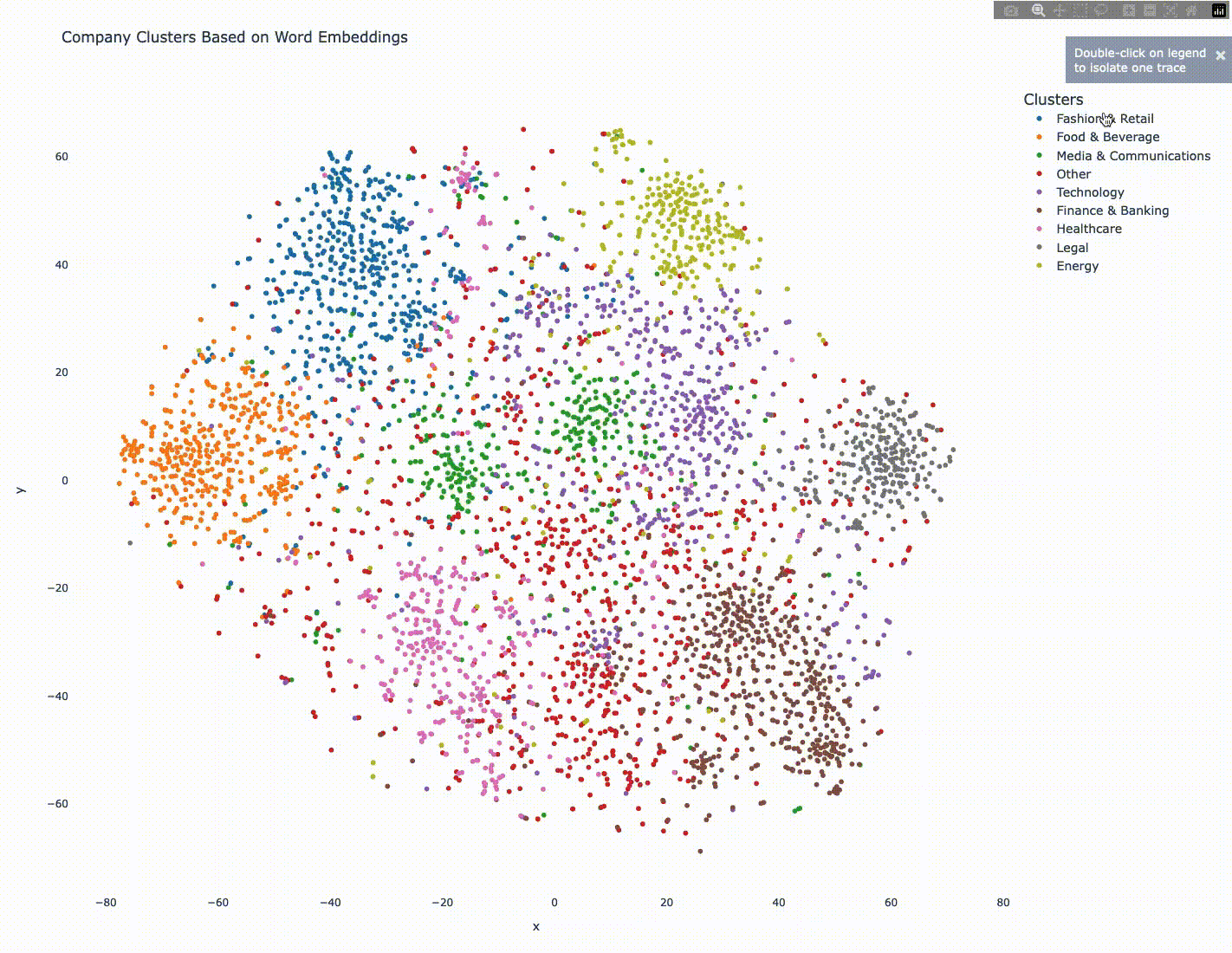
What we've done with artificial intelligence
Technical advances in AI are quickly catching up with CRE industry needs, with various solutions being built to track, aggregate, and link information across a tenant’s and a property’s lifecycle. From de-anonymization (the practice of linking anonymous data to publicly available information), to entity disambiguation (the task of assigning unique identities to disparate records), and word embeddings (the representation of words in high-dimensional vector space) - these technologies power the domain-specific AI solutions that VTS has built internally to connect the dots across billions of data points and thousands of unique client integrations.
Our data platform orchestrates flows of first-party data between our Lease Management, Market, Tenant Experience, and Data products so that we can derive insights not just on a single building, but also the relationships between them and understand the interaction between physical space and tenant behavior in the real world. For example, we can track for a unique tenant company:
- The number of repeat views they had for a vacant space online
- The physical tours they took across buildings
- The amenity reservation behavior of their employees
By focusing on training domain-specific ML models to do single tasks (like de-anonymization and disambiguation) really well, we’ve also unlocked the ability to produce unique insights about market supply and demand.
Take our tenant requirement disambiguation model, for example, which finds similar-looking deal logs that represent the same unique tenant touring in a specific market. The model leverages similar techniques to other AI applications, namely high dimensional vector embeddings, to find similar records across spatio-temporal attributes (in other words - deal opportunities logged in similar locations and at similar times with similar broker or company attributes). The model currently performs at 92%+ accuracy to human-labeled data, and is on average, 3% off in demand prediction numbers.
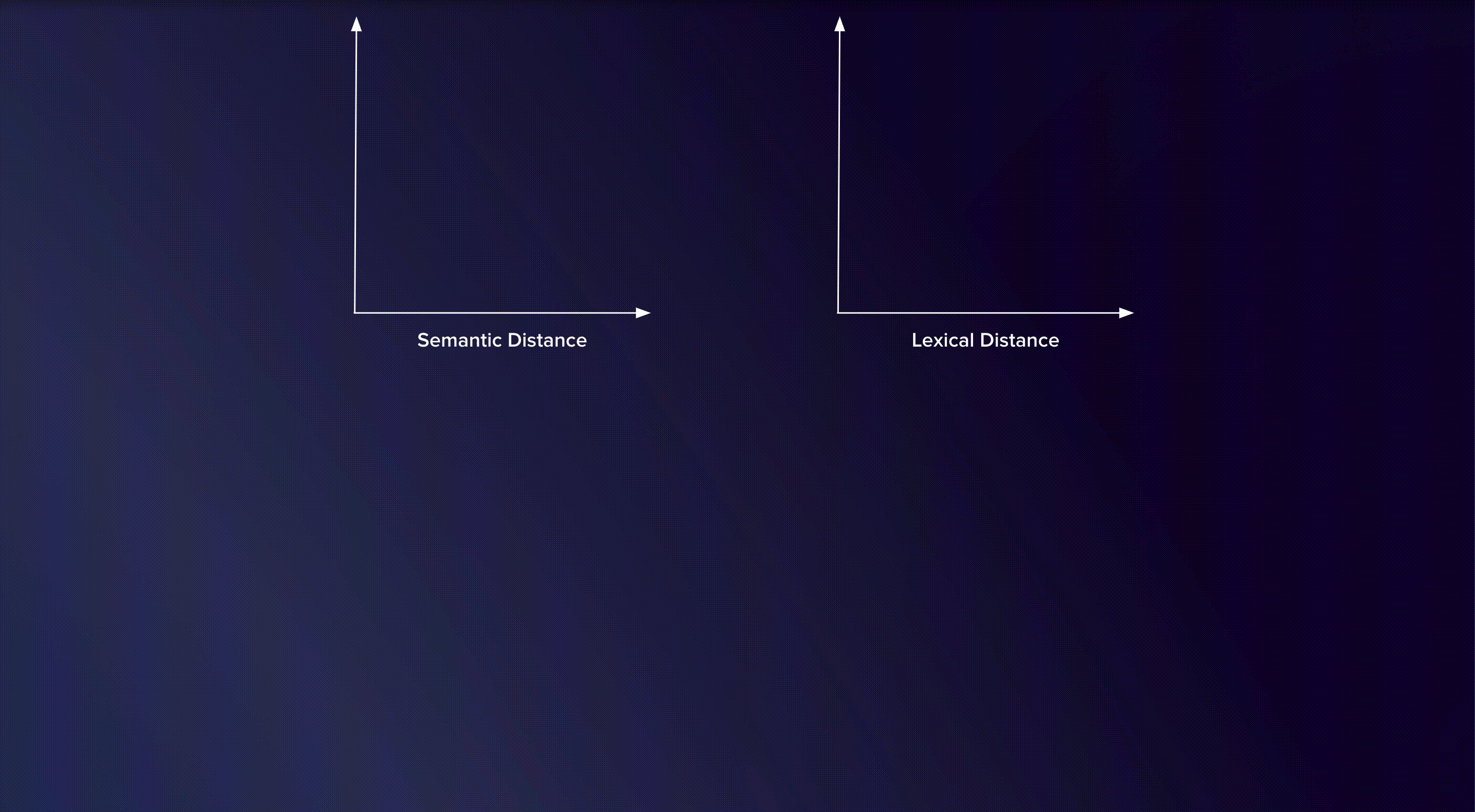
Diagrammatic representation of how our model finds relationships across deal logs, using semantic, lexical, and spatio-temporal distances—distances that are impervious to errors in data entry
This model is one element that powers our monthly VODI index and is part of the broader VTS Demand Model. We have employed similar techniques on company and property disambiguation tasks, whereby we match entities across thousands of systems to the same real world record. We do this by leveraging pre-trained word embeddings, and calculating a custom loss function to perform matching operations that overcome bad data entry and missing attributes (such as “space”, “condition”, “tenant name”, “tenant industry”).
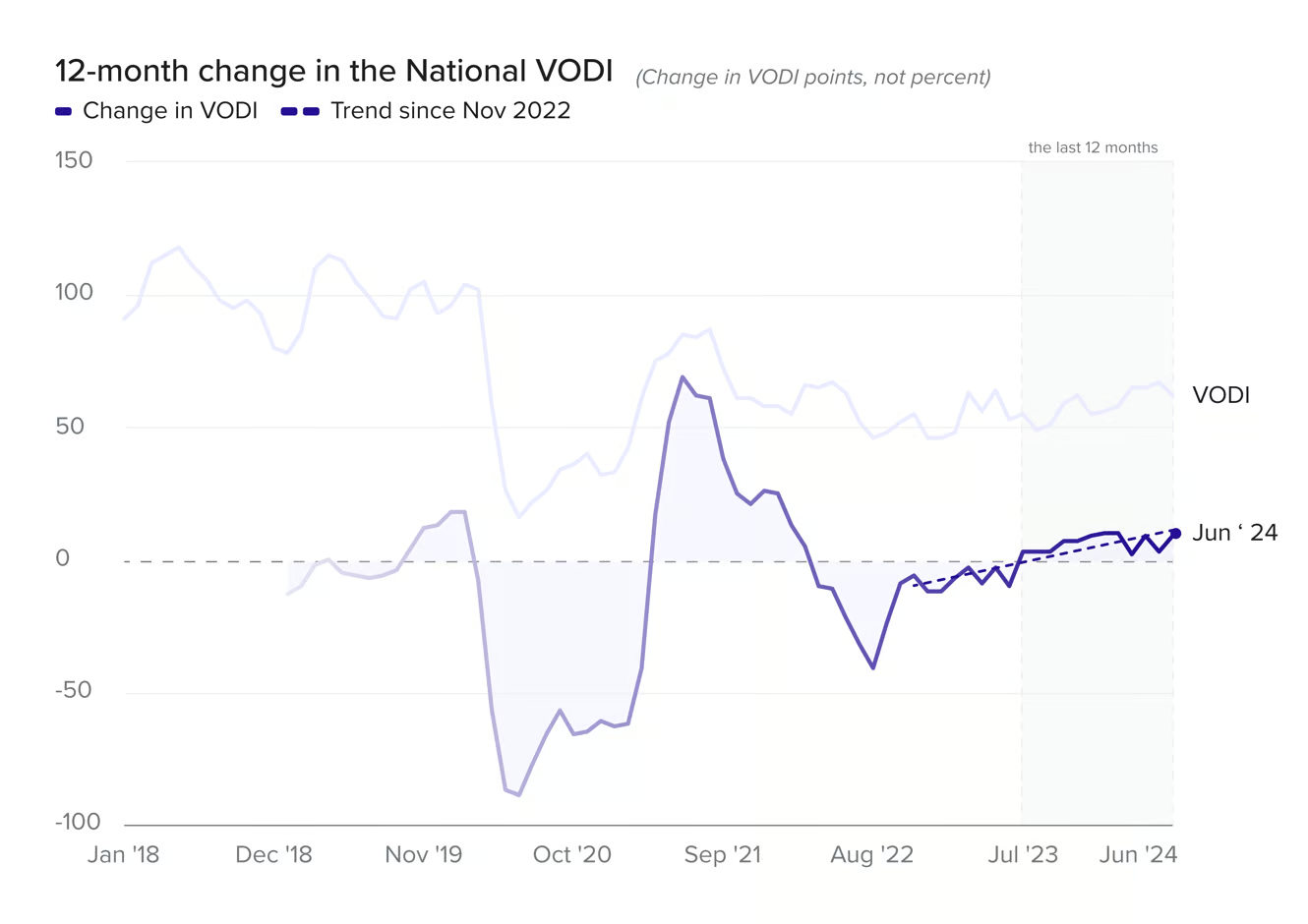
Snapshot of July 2024 VODI Index
What’s next
At the heart of our AI strategy is a desire to simplify real-world complexity. This started with piecing together fragmented data that reflects “IRL” activities—all to help our customers make their toughest decisions based on a real-time understanding of the market. Now it’s about simplifying IRL activities themselves.
Take managing work orders in a building, for instance. For property management, the complexity of filtering through work order requests, prioritizing which to fulfill, and sending the right help is complicated and time consuming. The risk of a slow response and fix has a real impact on tenant sentiment, especially if it’s a tenant at risk for renewal. By focusing the next stage of AI/ML on automating workflows like service requests, preventive maintenance, and more, the impact of AI will be tangible to both our owner/operator customers and their tenants.
Of course, our AI/ML team is also doing research and building generative AI applications to transform the customer experience. At VTS, we’re uniquely positioned to provide a GPT-like interface that can provide not just answers to deep questions about things like tenant risk and sentiment, but also provide logical predictions and probabilities of certain events occurring within an owner’s portfolio.
Let’s not lose sight of AI’s base requirement: People
We know real advances in commercial real estate will not come from technology alone. Researchers have been predicting that machine learning will disrupt the real estate industry for many years. We believe that process, culture, and a willingness to experiment have to be evolved hand-in-hand, which is why our Product, Engineering, and Design teams work tirelessly to create a seamless experience for us to capture, analyze and display information to various personas. Because for us to connect the dots the information has to be captured and monitored in the first place, by people in the real world. It is going to take the professionals in the industry as a whole to be committed to behavioral change around data collection and governance to realize the full potential of AI. But when it happens, the impact to everything from investment due diligence, revenue forecasting, underwriting, and pricing will be immense.
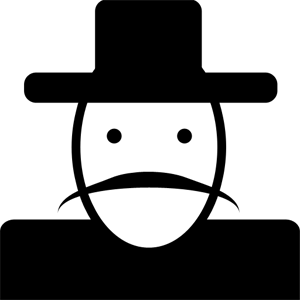